ArangoDB Newsletter #132: April Updates and Insights
Estimated reading time: 4 minutes
Hello Community,
Welcome to the fourth ArangoDB newsletter of 2021!
In this edition, we share details about: our latest and greatest lunch breaks, part four of our ArangoML blog series, as well as a guest article featured in DZone about the C++ memory model.
We hope you enjoy!
ArangoDB Newsletter #131: March Updates and Insights
Estimated reading time: 4 minutes
We’ve officially entered Spring this month, so perhaps it’s time for a little spring cleaning? Here’s a few suggestions from us:
- Optimize your AQL queries by checking out our latest lunch breaks
- Learn something new with our ArangoML blog series
Anything else you’d like to learn about? Drop us a line anytime at community [at] arangodb [dot] com.
ArangoDB Newsletter #130: February Updates and Insights
Estimated reading time: 4 minutes
Hello community!
This month we post on the blog bearing gifts up upcoming events, content we think you might enjoy, as well as suggestions around how to get involved with the community. Read on!
We’d also love to hear from *you*! Drop us a line anytime at community [at] arangodb [dot] com.
C++ Memory Model: Migrating from x86 to ARM
Estimated reading time: 18 minutes
Over the past years, we have seen a shift in processors from the previously dominant x86 architecture to the more energy-efficient (and often cheaper) ARM architecture. This trend is true for both consumer hardware, e.g., Apple’s M1 SoC and also server hardware, e.g. AWS’s Gravitron processor. Given that the ARM architecture has a more relaxed memory model, this might have some subtle impact on C++ programs…
With the introduction of multi-threaded execution in C++11, the standard also introduced a memory model. For many developers, this memory model is one of the least well-understood parts of the C++ standard and a common source of confusion. While an in-depth explanation of the C++ memory model is beyond this blog post’s scope, we will cover some basics and take a brief look at two of the most common hardware memory models and how the C++ memory model maps to these hardware models.
But first, let’s take a step back and consider why we need a memory model in the first place.
(more…)ArangoDB Newsletter #129: Stay Informed with the Latest Updates
Estimated reading time: 4 minutes
Happy New Year to our amazing community!
We hope your year is off to a good start. Even though things don’t seem too different (yet), we’re definitely feeling a fresh mindset and doing our best to stay hopeful and healthy.
Here’s what we’re excited about so far:
- Our new ‘Graph & Beyond’ Lunch Break series
- An upcoming webinar about Graph Analytics
- Our ArangoML blog series
Read on for more!
ArangoML Series: Multi-Model Collaboration
Estimated reading time: 8 minutes
Multi-Model Machine Learning
This article looks at how a team collaborating on a real-world machine learning project benefits from using a multi-model database for capturing ML meta-data.
The specific points discussed in this article are how:
- The graph data model is superior to relational for ML meta-data storage.
- Storing ML experiment objects is natural with multi-model.
- ArangoML promotes collaboration due to the flexibility of multi-model.
- ArangoML provides ops logging and performance analysis.

State of the Art Preprocessing and Filtering with ArangoSearch
Estimated reading time: 10 minutes
Just in case you haven’t heard about ArangoSearch yet, it is a high-performance Full-Text Search engine integrated in ArangoDB (meaning connected with the other data-models and AQL). Feel free to check out ArangoSearch – Full-text search engine including similarity ranking capabilities for more details.
In ArangoDB version 3.7 the ArangoSearch team added Fuzzy Search support (see the comprehensive article Fuzzy search by Andrey Abramov). With Fuzzy Search data preprocessing and filtering becomes even more important. In the upcoming ArangoDB 3.8 release, ArangoSearch efforts will be focused on improving this part. In this post I’m going to uncover some of the new features we are proud to present.
(more…)ArangoML Series: Intro to NetworkX Adapter
Estimated reading time: 3 minutes
This post is the fifth in a series of posts introducing the ArangoML features and tools. This post introduces the NetworkX adapter, which makes it easy to analyze your graphs stored in ArangoDB with NetworkX.
In this post we:
- Briefly introduce NetworkX
- Explore the IMDB user rating dataset
- Showcase the ArangoDB integration of NetworkX
- Explore the centrality measures of the data using NetworkX
- Store the experiment with arangopipe
This notebook is just a slice of the full-sized notebook available in the ArangoDB NetworkX adapter repository. It is summarized here to better fit the blog post format and provide a quick introduction to using the NetworkX adapter.
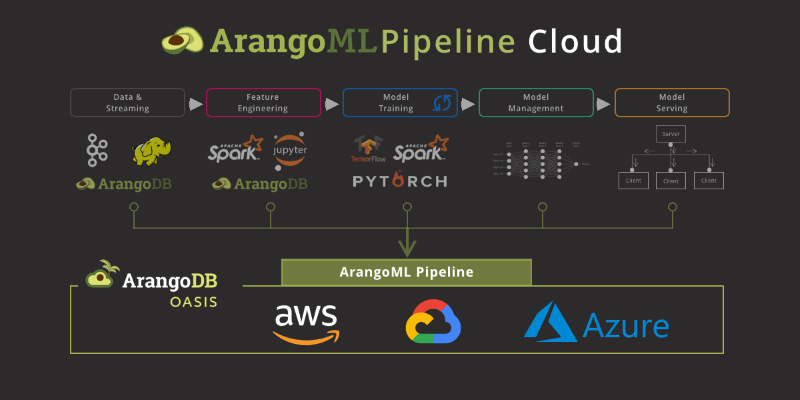
ArangoML Part 4: Detecting Covariate Shift in Datasets
Estimated reading time: 1 minute
This post is the fourth in a series of posts introducing ArangoML and showcasing its benefits to your machine learning pipelines. Until now, we have focused on ArangoML’s ability to capture metadata for your machine learning projects, but it does much more.
In this post we:
- Introduce the concept of covariate shift in datasets
- Showcase the built-in dataset shift detection API

ArangoML Part 3: Bootstrapping and Bias-Variance
Estimated reading time: 2 minutes
This post is the third in a series of posts about machine learning and showcasing the benefits ArangoML adds to your machine learning pipelines. In this post we:
- Introduce bootstrapping and bias-variance concepts
- Estimate and analyze the variance of the model from part 2
- Capture the metadata for this activity with arangopipe
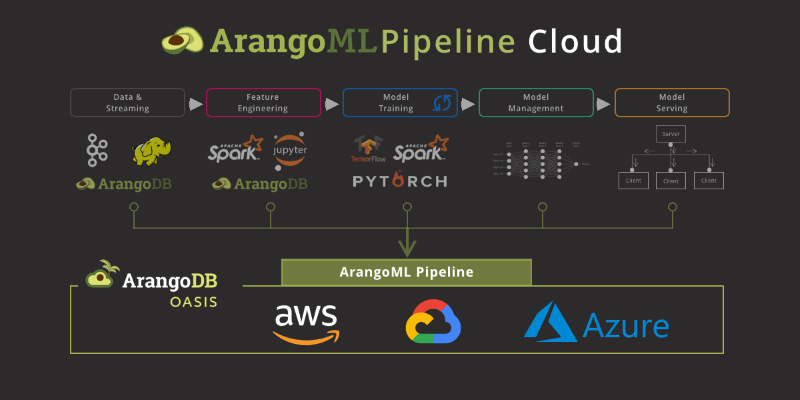
Get the latest tutorials,
blog posts and news:
Thanks for subscribing! Please check your email for further instructions.