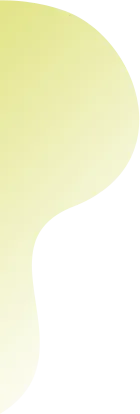



Get started free today
(no credit card required), and experience the shortest time to value for a hosted graph DB.

Download the Scalable Fraud Detection White Paper
Get a how-to guide for building a Fraud Detection solution with Graph.

Enter Entity Resolution
Entity resolution is crucial for merging similar entities from different sources into one record, essential for consolidating numerous deceptive accounts. ArangoDB stands out in Entity Resolution, processing various data types simultaneously to reveal hidden correlations and patterns, essential for detecting sophisticated fraudulent activities with unmatched precision and efficiency. This is only made possible by ArangoDB's multi-model architecture.
Relating Disparate Fraud Data Points
Specifically, Entity Resolution is key in fraud detection by linking diverse data (IP addresses, emails, and device authentication methods, etc.) to uncover fraudulent activity. This technique detect fraudsters, unveils their true identities, and tracks their activities across various accounts and platforms, bolstering defenses against fraudulent activities.


Real-Time Fraud Detection
Real-time graph analytics can empower fraud detection systems with low-latency queries, enabling timely analysis of incoming data streams and rapid identification of suspicious patterns. This can be challenging to achieve using legacy graph databases, and even moreso with traditional RDBMS systems. ArangoDB's automatic query optimizations significantly reduce query latency, another unique differentiator.
Machine Learning
Integration
ArangoDB not only seamlessly integrates with machine learning frameworks to enable sophisticated fraud detection models. ArangoGraphML itself provides advanced Graph ML algorithms to enhance the accuracy and predictive power of fraud detection techniques.


Collaborative Filtering
Uncover fraudulent user behavior with ArangoDB's graph-based collaborative filtering. Analyze user interactions, relationships, and patterns, recommending potential fraudsters or anomalies that may go undetected with typical graph databases and RDBMS-based systems.


ArangoDB vs. Legacy Graph DBs
For Fraud Detection
![]() | ArangoDB For Fraud Detection | Neo4j & Others for Fraud Detection |
---|---|---|
Native Multi-Model Support | ![]() | ![]() |
Foxx Microservices | ![]() | ![]() |
Built-in Search (with unified query language) | ![]() | ![]() |
SmartGraphs | ![]() | ![]() |
Cross-Collection Joins | ![]() | ![]() |

Get started free today
(no credit card required), and experience the shortest time to value for a hosted graph DB.

Download the Scalable Fraud Detection White Paper
Get a how-to guide for building a Fraud Detection solution with Graph.