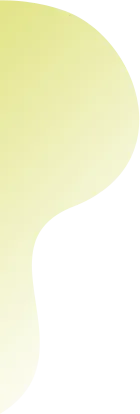
The Transformative Power of ArangoDB GraphRAG in Genomics-Driven Personalized Medicine
Estimated reading time: 7 minutes
Introduction
Personalized medicine is a truly disruptive innovation in healthcare. Medical treatment can now pivot from mass-market, standardized care models to custom-made, client-centric solutions. For example, healthcare providers can now offer precision-targeted therapeutic products and services using individual genetic data and lifestyle metrics. And this shift would drive improved patient outcomes. It would also open up brand-new market segments and revenue streams for healthcare organizations. Selling commoditized therapies turns into offering customized data-driven health management.
Personalized medicine challenges existing business models for stakeholders across the healthcare ecosystem. From pharmaceutical companies to insurers and care providers, personalized medicine becomes an opportunity for competitive differentiation.
In the long term, personalized medicine has the potential to redefine the entire healthcare value chain. New touchpoints for customer engagement emerge. Providers could cultivate a more proactive approach to health management. These therapies could be based on individual genetic profiles, environmental factors, and lifestyle choices.
At the intersection of cutting-edge genomics and advanced computational methods lies an opportunity to revolutionize patient care through technologies like GraphRAG (Graph Retrieval Augmented Generation). This white paper explores how ArangoDB's GraphRAG implementation offers unique capabilities for tackling complex challenges in personalized medicine, presenting high-impact applications with detailed analyses of challenges, solutions, and potential returns on investment.
Knowledge graphs now converge with vector-based search methods. Healthcare providers can extract meaningful insights from complex, diverse, interconnected medical data. The goal is to deliver far more accurate diagnoses and efficacious treatments. Enhanced patient outcomes will emerge across a range of clinical scenarios.
The Promise and Challenges of Personalized Medicine
"The ability to sequence an entire human genome for less than the cost of a chest x-ray series has changed everything. We are entering an era where we will be able to provide truly personalized care based on an individual's genetic makeup. However, we are still in the infancy of understanding how to interpret and apply this vast amount of information."
-Dr. Francis Collins, former director of the National Institutes of Health, key figure in the Human Genome Project.
The human genome project's completion in 2003 promised a new era of medicine where treatments would be precisely calibrated to an individual's genetic makeup. Yet, two decades later, we still struggle to realize this vision fully. Why? Because biological systems are fiendishly complex, and the tools to navigate this complexity have been, until recently, woefully inadequate.
The challenge isn't a lack of data—quite the opposite. Modern healthcare systems are drowning in information: electronic health records, genome sequencing data, biomarker measurements, clinical trial results, and a constant torrent of new research findings. What's missing is the ability to connect these disparate data points in meaningful ways, to extract insights from the noise, and to present these insights in a format that supports clinical decision-making.
Traditional databases struggle with this task because they weren't designed to handle the inherently interconnected nature of biological and medical knowledge. Relational databases force complex relationships into rigid tables, while document stores lack the structure to navigate connections efficiently. Vector databases can capture semantic similarities but miss critical relationship context.
This is where graph databases—and specifically ArangoDB's GraphRAG technology—enter the picture. By combining the relationship-focused power of knowledge graphs with the semantic capabilities of vector embeddings, GraphRAG offers a powerful new approach to personalized medicine challenges. The integration of large language models (LLMs) with knowledge graphs creates a system that retrieves relevant information and generates contextually appropriate insights and recommendations.
GraphRAG: Where Vector Retrieval meets Knowledge Graphs
Let's clarify what makes GraphRAG unique before diving into specific applications. Traditional Retrieval Augmented Generation (RAG) typically relies on vector embeddings to find content that is semantically similar. While effective for many applications, this approach treats documents as isolated units, missing the rich web of relationships between entities.
By contrast, GraphRAG structures information as interconnected nodes and edges in a knowledge graph. This allows for precise traversal of relationships—critical in medical contexts where understanding how entities relate to each other is often more important than finding similar text.
When a doctor asks, "What treatments are effective for patients with this genetic variant?" they're not looking for semantically similar documents; they're asking for a specific traversal of the relationship between variants, conditions, and treatments.
ArangoDB's implementation takes this a step further by offering a multi-model database that combines the power of graph, document, and key-value structures in a single platform. This flexibility is particularly valuable in healthcare scenarios where different types of data—structured, semi-structured, and unstructured—must be integrated seamlessly.
The integration of graphs with LLMs adds another dimension. Natural language queries are translated into precise graph traversals, with the results contextualized and presented in human-readable form. Bidirectional translation—from natural language to graph queries and back—makes the system accessible to clinical users without requiring expertise in graph query languages.
Now, let's take a look at a couple of high-impact applications of GraphRAG in personalized medicine.
Application 1: Pharmacogenomics-Based Drug Selection
The Challenge
A patient's response to medications varies dramatically based on their genetic makeup. A drug that works perfectly for one patient might be ineffective or even dangerous for another due to variations in genes that encode drug-metabolizing enzymes, transporters, or target receptors. The field of pharmacogenomics addresses this variability, but implementing its insights in clinical practice remains difficult.
Let’s take Warfarin, a widely used blood thinner, as a prime example of our current data integration challenge. Proper dosing of this medication is critical, with a razor-thin margin between an ineffective dose and one that could cause dangerous bleeding.
Our current systems struggle to efficiently integrate key data:
- Standard dosing protocols
- Characteristics of individual patients
- Genetic markers that can influence drug metabolism
Specifically, variations in two genes - CYP2C9 and VKORC1 - could require dose adjustments of up to 80% from standard protocols Without a system that can automatically flag these genetic variants and calculate adjusted dosing, we're leaving our clinicians to manually juggle complex data sets, increasing both cognitive load and the risk of errors. Similar challenges exist for numerous medications across therapeutic areas.
Today, healthcare providers face several obstacles when trying to incorporate pharmacogenomic insights. As discussed earlier, the knowledge base is vast and evolves rapidly, with new gene-drug interactions published weekly. And the relevant information is scattered across databases, research papers, and clinical guidelines. Interpreting the clinical significance of specific genetic variants also requires highly specialized expertise. Finally, integrating pharmacogenomic data with other clinical factors (age, organ function, co-medications) adds much complexity for clinicians.
Possible Solutions
Several approaches exist to address these challenges:
- Standalone pharmacogenomic decision support systems: These specialized tools focus exclusively on gene-drug interactions but often lack integration with broader clinical data
- Vector-based RAG systems: These can retrieve relevant literature based on semantic similarity but struggle with the precise relationship mapping needed for pharmacogenomic recommendations
- Rule-based expert systems: These encode explicit if-then rules for pharmacogenomic guidelines but are difficult to maintain as knowledge evolves
- ArangoDB's GraphRAG-type approach: This combines structured knowledge representation with flexible retrieval and natural language generation
Why ArangoDB GraphRAG excels for drug selection based on pharmaco-genomics
ArangoDB's GraphRAG offers distinct advantages for pharmacogenomic applications. It allows for powerful capabilities that traditional systems struggle to achieve. Multi-hop reasoning becomes possible. The system is able to connect genetic variants to enzymes to drugs to alternatives in a single query. With ArangoDB's GraphRAG, you can now traverse complex relationships, a significant improvement over conventional methods.
We are also able to preserve context. In a knowledge graph, the relationships between entities, such as how exactly a drug affects a genetic variant, are explicitly represented. Therefore, with a graph, we ensure that we don't lose crucial contextual information during the reasoning process.
Using ArangoDB's GraphRAG also allows us to integrate multiple data types. We can now cohesively query across structured variant data, unstructured clinical guidelines, and semi-structured patient records. This ability to seamlessly work with diverse data formats is crucial in the rather complex landscape of healthcare data management.
Dynamic knowledge updates become possible with ArangoDB's GraphRAG.
In the rapidly evolving field of genetics, there are novel pharmacogenomic findings emerging weekly. The ArangoDB knowledge graph could be updated without the need to retrain the entire system. This flexibility ensures that the system stays current with the latest scientific discoveries, providing up-to-date insights for clinical decision-making.
Imagine a system that transforms how clinicians interact with patient data.
Instead of manually cross-referencing multiple databases and guidelines, they could simply ask a question in natural language:
"What antidepressants are recommended for this patient given their CYP2D6 gene's poor metabolizer status?"
A platform built with ArangoDB's GraphRAG would access the data, query using AQL, and integrate data from multiple sources to answer this question. This would include the patient's electronic health record, the hospital's pharmacogenomic database, up-to-date clinical guidelines, and the latest research literature. We would get a comprehensive response from the platform's advanced analytics.
This response would include a prioritized list of recommended medications, along with an underlying reason for each recommendation, that takes into consideration the patient's specific genetic profile. It would also highlight potential drug interactions based on the patient's current medications and suggest appropriate dosing adjustments.
All of this information would be presented in a clear, actionable format for the clinician. Prescription errors would go down and the efficacy of therapies could be improved dramatically. We could really streamline clinical workflows.
We see a shift from passive data storage to active clinical decision support. This potentially reduces adverse drug events and associated costs while improving patient outcomes. Moreover, this system would be scalable across various medical specialties and adaptable as new genetic insights emerge, providing long-term value for the healthcare organization.
ROI Comparison
Implementing pharmacogenomic guidance through different approaches yields varying returns:
- Vector-only RAG: Can improve information retrieval but lacks the precision for clear recommendations, resulting in a modest 20-25% improvement in appropriate prescribing.
- ArangoDB GraphRAG: By combining precise relationship traversal with natural language interaction, adoption rates rise to 40-60%, with corresponding improvements in outcomes. One healthcare system reported an annual savings of $2.2M after implementing a GraphRAG-based pharmacogenomics approach.
The GraphRAG approach provides clear, contextual guidance that physicians can trust and easily incorporate into their workflow. This offers a significant ROI advantage over Vector-only RAG.
Application 2: Disease Risk Prediction and Prevention
The Challenge
You need to integrate multiple data types to predict an individual's risk for complex diseases like Alzheimer's, diabetes, cancer, or heart disease. These include genetic risk variants, family history, environmental exposures, lifestyle factors, and biomarker measurements. Traditional risk calculators use simplified models that capture only a fraction of these interactions, while more sophisticated approaches often become "black boxes" that clinicians hesitate to trust.
The challenges are many:
- Risk factors interact in complex, non-linear ways that simple scoring systems can't capture
- Different risk factors operate on different time scales and with varying degrees of certainty
- Preventive interventions need to be tailored to the specific combination of risk factors
- Explaining risk assessments in an understandable way to clinicians is crucial for patient engagement
"We had a patient with a strong family history of breast cancer, but no identifiable BRCA1 or BRCA2 mutation. Her Tyrer-Cuzick risk score was only slightly elevated. But when we looked at her polygenic risk score, incorporating multiple moderate-risk variants, it put her at much higher risk. This case really highlighted for me how our traditional risk models might be missing important genetic contributions to cancer risk."
- Dr. Judy Garber, Director of the Center for Cancer Genetics and Prevention at Dana-Farber Cancer Institute, speaking at 2019 San Antonio Breast Cancer Symposium.
Dr. Garber's comment clearly demonstrates the limitations of conventional approaches.
Possible Solutions
We could approach the challenge in different ways:
- Statistical risk models: Frameworks like Framingham Risk Score or BOADICEA use statistical methods to combine risk factors, but they handle only a limited set of variables
- Machine learning models: These can capture complex interactions but often function as black boxes, making explanation difficult.
- Vector database approaches: These can retrieve similar cases but struggle to provide the causal reasoning needed to plan the intervention on the patient.
- ArangoDB GraphRAG-type systems: These actually represent the causal relationships between risk factors, diseases, and interventions, enabling the clinician to both predict and explain.
Why ArangoDB GraphRAG Excels
ArangoDB's GraphRAG approach is uniquely suited to disease risk prediction. For example, you would build a query in AQL that is able to navigate through various types of risk factors, such as genetic risks, lifestyle risks, environmental risks and biomarker risks.
There are several advantages to this approach with ArangoDB’s GraphRAG. Firstly, we can represent causal relationships, not just correlation.
The knowledge graph explicitly represents causal relationships between risk factors and diseases, enabling explanation rather than just predictions! Next, multi-modal integration is now possible with ArangoDB's GraphRAG approach. Genetic, environmental, and clinical data are integrated in a single model that preserves their relationships.
The clinician could plan for personalized patient interventions. The system can recommend interventions to the clinician targeted at the specific risk factors identified for an individual patient. Finally, the graph structure allows us to generate natural language explanations that trace the path from risk factors to disease risk to interventions.
ROI Comparison
Different approaches to disease risk prediction yield varying economic returns, based on implementations and studies:
- Traditional risk calculators: These improve risk stratification by 15-20% over clinical judgment alone, leading to modest improvements in preventive care utilization and an ROI of approximately 1.5:1.
- ML-based models: These can improve prediction accuracy by 25-35% but face adoption challenges due to explainability issues to clinicians, resulting in an ROI of 2:1 when successfully implemented.
- Vector-only approaches: These improve information retrieval but struggle with the causal reasoning needed for intervention planning by clinicians, limiting ROI to around 1.8:1.
- ArangoDB's GraphRAG-type approach: By combining accurate risk prediction with explainable reasoning and targeted intervention recommendations, this approach has demonstrated ROI ratios of 3:1 to 4:1 in new implementations.
The superior ROI of GraphRAG comes from its ability to identify who is at risk but also explain why they're at risk. More importantly, it goes into what specifically can be done about it. Clinicians can now implement preventive interventions tailored to individual risk profiles!
The Future of ArangoDB's GraphRAG in Personalized Medicine
The applications described in this white paper represent just the beginning of what's possible with ArangoDB's GraphRAG technology in personalized medicine. As healthcare continues to generate more data across modalities—genomics, proteomics, metabolomics, digital biomarkers, imaging, and electronic health records—the need for systems that can integrate and reason across these data types will only grow.
We see that ArangoDB's GraphRAG technology offers a powerful approach to these challenges by combining the strengths of knowledge graphs, vector embeddings, and large language models. The multi-model nature of ArangoDB's graph database and its query language AQL, is particularly well-suited to the heterogeneous data landscape of healthcare, while the integration with natural language processing makes the system accessible to clinical users without specialized technical expertise.
Looking ahead, we can anticipate several trends in the evolution of GraphRAG for personalized medicine:
- Increasingly automated knowledge graph construction: Tools that can automatically extract entities and relationships from the biomedical literature, reducing the manual curation burden
- Multimodal integration: Incorporation of imaging data, sensor readings, and other non-textual modalities into the knowledge graph
- Temporal reasoning: Enhanced capabilities for reasoning about changes over time, crucial for understanding disease progression and treatment response.
- Distributed knowledge graphs: Federation across institutions to enable larger, more comprehensive knowledge structures while preserving privacy and governance.
As these technologies mature, the vision of truly personalized medicine—tailored not just to broad population groups but to each individual's unique biological, clinical, and environmental context—comes closer to reality. GraphRAG technologies like those offered by ArangoDB represent a crucial step toward that future, offering healthcare providers powerful tools to navigate the complexity of human biology and deliver more precise, effective care.
References
- ArangoDB. (2024). GraphRAG - ArangoDB. Retrieved from https://arangodb.com/graphrag/
- Yu, PhD MD. (2024, September 14). How GraphRAG Can Enhance Healthcare: Improving Medical... LinkedIn. Retrieved from https://www.linkedin.com/pulse/how-graphrag-can-enhance-healthcare-improving-medical-yu-phd-md--fk7ae
- E2E Networks. (2025, February 27). Healthcare Knowledge Graph RAG with Neo4j - E2E Networks. Retrieved from https://www.e2enetworks.com/blog/building-a-healthcare-knowledge-graph-rag-with-neo4j-langchain-and-llama-3
- ArangoDB Documentation. (2013, November 3). Example graphs | ArangoDB Documentation. Retrieved from https://docs.arangodb.com/3.11/graphs/example-graphs/
- Gradient Flow. (2024, August 15). GraphRAG Meets Finance: Enhancing Unstructured Data Analysis... Retrieved from https://gradientflow.com/graphrag-nvidia-blackrock/
- Santosa, A. (2024, August 20). The Role of GraphRAG in Modern Healthcare Systems. LinkedIn. Retrieved from https://www.linkedin.com/pulse/role-graphrag-modern-healthcare-systems-anindita-santosa-5rqxc
- YouTube. (2024, September 23). ArangoDB GraphRAG Technical Demo - YouTube. Retrieved from https://www.youtube.com/watch?v=2Izn5g22m_0
- ArangoDB. (2024, December 2). Data Science Suite Page - ArangoDB. Retrieved from https://arangodb.com/data-science-suite-page/
- Lu, S., & Cosgun, E. (2024, November 15). Boosting GPT Models for Genomics Analysis: Generating Trusted Genetic Variant Annotations and Interpretations through RAG and fine-tuning. bioRxiv. Retrieved from https://www.biorxiv.org/content/10.1101/2024.11.12.623275v1.full.pdf
- ArangoDB. (2024, November 26). Jupyter Notebooks - ArangoDB. Retrieved from https://arangodb.com/jupyter-notebooks/
- Data Graphs. (2025, January 1). Unlock Smarter Insights with GraphRAG AI - Data Graphs. Retrieved from https://datagraphs.com/use-cases/graphrag-ai
- ArangoDB. (2024, August 5). Decoded Health | Transforming Healthcare with ArangoDB. Retrieved from https://arangodb.com/solutions/case-studies/decoded-health-transforming-healthcare-with-ml-models-ontologies-and-graphs/
- Prism14. (2024, September 26). Top 3 Applications of GraphRAG Systems Across Different Fields. Retrieved from https://prism14.com/top-3-applications-of-graphrag-systems-across-different-fields/
Get the latest tutorials, blog posts and news: