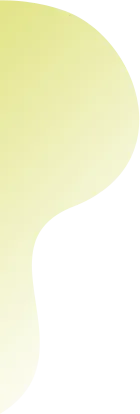


Get started free today
(no credit card required), and experience the shortest time to value for a hosted graph DB.

Read the
Case Studies
Learn why companies across industries are switching to ArangoDB for Graph.

Hybrid Recommendations
By leveraging content-based and collaborative filtering, ArangoDB uses content-based and collaborative filtering to match user preferences with product attributes, delivering precise recommendations. By combining user and product data, it provides personalized suggestions in milliseconds, making it ideal for e-commerce platforms looking to enhance user engagement.

Collaborative Filtering
Combining graph and document data models enhances collaborative filtering. The graph model maps user and item relationships, uncovering complex interaction patterns for accurate recommendations. The document model stores detailed user and item attributes, offering deep insights into individual preferences. Real-time integration of these models enables highly personalized and precise recommendations.


Real-Time Personalization
ArangoDB, with its fast data processing and AQL, adjusts recommendations in real-time to match user behavior. For streaming, it instantly tailors content suggestions to evolving viewing habits. This is made possible by ArangoDB’s multi-model approach, which quickly connects user and content data for a responsive, personalized experience.
Contextual Recommendations
ArangoDB uses geo-spatial indexing and time-based queries to provide contextual recommendations by analyzing location and time data. For travel, the platform merges varied datasets to suggest nearby attractions and events based on the user’s current location and time. By supporting diverse data types in one platform, ArangoDB ensures relevant, context-aware suggestions.



Scalable Recommendation System
ArangoDB's cluster scalability allows recommendation systems to manage large user bases and growing data volumes. In ride-sharing, this enables real-time driver-passenger matching and handles fluctuating demand efficiently.
ArangoDB vs. Legacy Graph DBs
For Recommendation Engines
![]() | ArangoDB For Recommendation Engines | Neo4j & Others for Recommendation Engines |
---|---|---|
Dynamic Schema Design | ![]() | ![]() |
Advanced Graph Algorithms | ![]() | ![]() |
Smart Indexing Optimization | ![]() | ![]() |
Cluster-Ready Scalability | ![]() | ![]() |
Streamlined Master Data Management | ![]() | ![]() |

Get started free today
(no credit card required), and experience the shortest time to value for a hosted graph DB.

Read the
Case Studies
Learn why companies across industries are switching to ArangoDB for Graph.